library(metan)
library(rio)
# gerar tabelas html
print_tbl <- function(table, digits = 3, ...){
knitr::kable(table, booktabs = TRUE, digits = digits, ...)
}
# dados
df_g <- import("http://bit.ly/df_g", setclass = "tbl")
inspect(df_g, verbose = FALSE) %>% print_tbl()
Variable |
Class |
Missing |
Levels |
Valid_n |
Min |
Median |
Max |
Outlier |
GEN |
character |
No |
0 |
39 |
NA |
NA |
NA |
NA |
BLOCO |
character |
No |
0 |
39 |
NA |
NA |
NA |
NA |
ALT_PLANT |
numeric |
No |
- |
39 |
1.81 |
2.27 |
3.04 |
0 |
ALT_ESP |
numeric |
No |
- |
39 |
0.75 |
1.19 |
1.88 |
0 |
COMPES |
numeric |
No |
- |
39 |
12.50 |
15.16 |
17.94 |
0 |
DIAMES |
numeric |
No |
- |
39 |
44.71 |
48.21 |
53.74 |
0 |
COMP_SAB |
numeric |
No |
- |
39 |
23.85 |
27.63 |
33.02 |
0 |
DIAM_SAB |
numeric |
No |
- |
39 |
13.28 |
15.88 |
18.28 |
0 |
MGE |
numeric |
No |
- |
39 |
105.72 |
169.05 |
236.11 |
0 |
NFIL |
numeric |
No |
- |
39 |
13.20 |
16.00 |
18.00 |
0 |
MMG |
numeric |
No |
- |
39 |
226.60 |
332.56 |
451.68 |
0 |
NGE |
numeric |
No |
- |
39 |
354.00 |
493.60 |
674.40 |
3 |
Modelo misto
A função gamem()
pode ser usada para analisar experimentos únicos(experimentos unilaterais) usando um modelo de efeito misto de acordo com o seguinte modelo:
$$
y_{ij} = \mu + \alpha_i + \tau_j + \varepsilon_ {ij}
$$
onde \(y_ {ij}\) é o valor observado para o \(i\)-ésimo genótipo no \(j\)-ésimo bloco (\(i\) = 1, 2, … \(g\); \(j\) = 1, 2,…, \(r\)); sendo \(g\) e \(r\) o número de genótipos e blocos, respectivamente; \(\alpha_i\) é o efeito aleatório do genótipo \(i\); \(\tau_j\) é o efeito fixo do bloco \(j\); e \(\varepsilon_ {ij}\) é o erro aleatório associado a \(y_{ij}\). Neste exemplo, usaremos os dados de exemplo df_g
.
gen_mod <-
gamem(df_g,
gen = GEN,
rep = BLOCO,
resp = everything())
## Evaluating trait ALT_PLANT |==== | 10% 00:00:00
Evaluating trait ALT_ESP |======== | 20% 00:00:00
Evaluating trait COMPES |============ | 30% 00:00:00
Evaluating trait DIAMES |================ | 40% 00:00:00
Evaluating trait COMP_SAB |=================== | 50% 00:00:00
Evaluating trait DIAM_SAB |======================= | 60% 00:00:00
Evaluating trait MGE |============================== | 70% 00:00:00
Evaluating trait NFIL |================================== | 80% 00:00:00
Evaluating trait MMG |======================================= | 90% 00:00:00
Evaluating trait NGE |===========================================| 100% 00:00:01
## Method: REML/BLUP
## Random effects: GEN
## Fixed effects: REP
## Denominador DF: Satterthwaite's method
## ---------------------------------------------------------------------------
## P-values for Likelihood Ratio Test of the analyzed traits
## ---------------------------------------------------------------------------
## model ALT_PLANT ALT_ESP COMPES DIAMES COMP_SAB DIAM_SAB MGE
## Complete NA NA NA NA NA NA NA
## Genotype 2.27e-12 2.36e-13 0.000224 5.95e-07 9.69e-09 0.000311 4.67e-08
## NFIL MMG NGE
## NA NA NA
## 0.00145 8.3e-08 0.00907
## ---------------------------------------------------------------------------
## All variables with significant (p < 0.05) genotype effect
A maneira mais fácil de obter os resultados do modelo acima é usando a função gmd()
, ou seu shortcut gmd()
.
Diagnósticos do modelo
plot(gen_mod, type = "res") # padrão
## `geom_smooth()` using formula 'y ~ x'
## `geom_smooth()` using formula 'y ~ x'
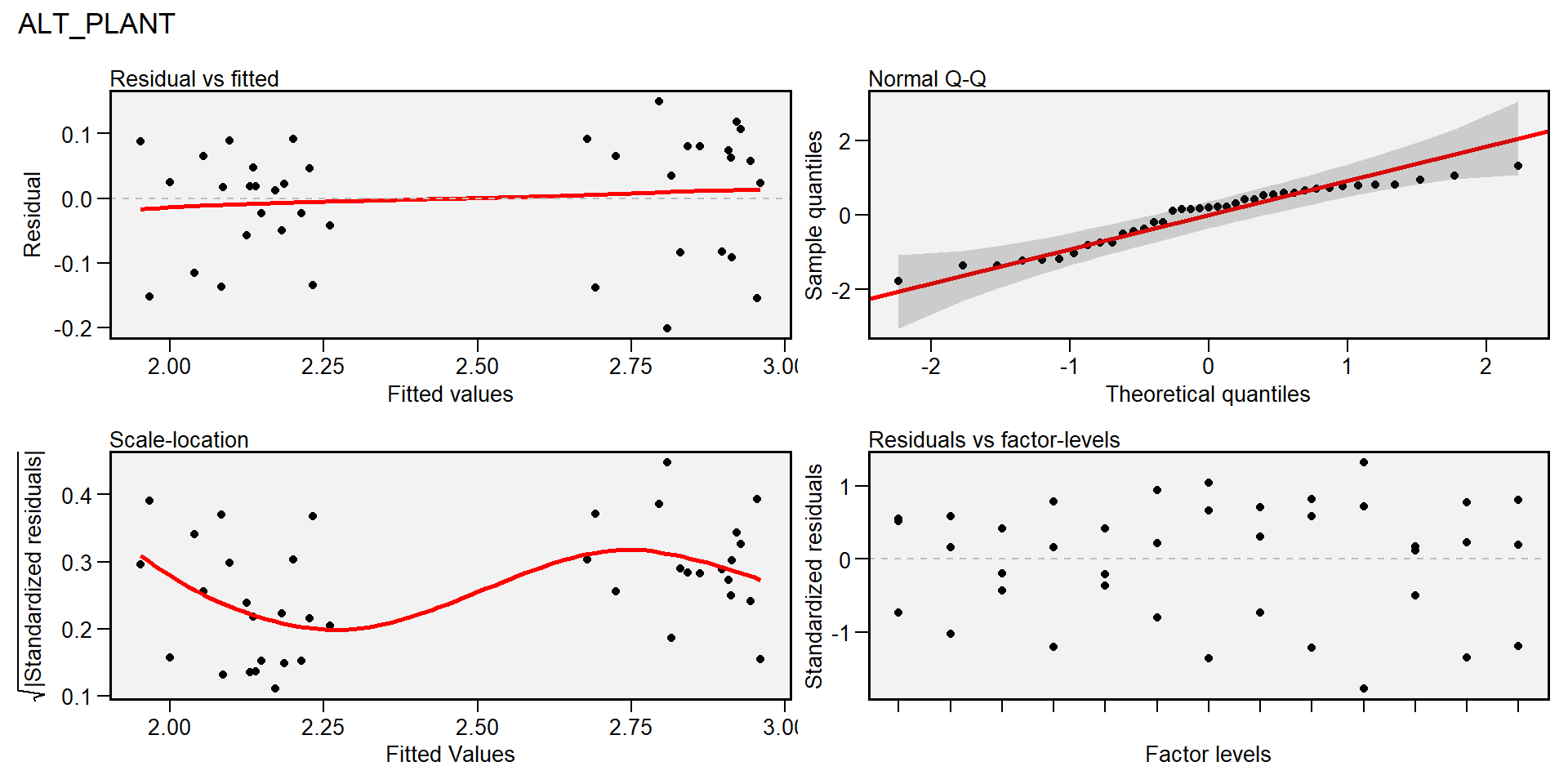
plot(gen_mod, type = "re") # padrão
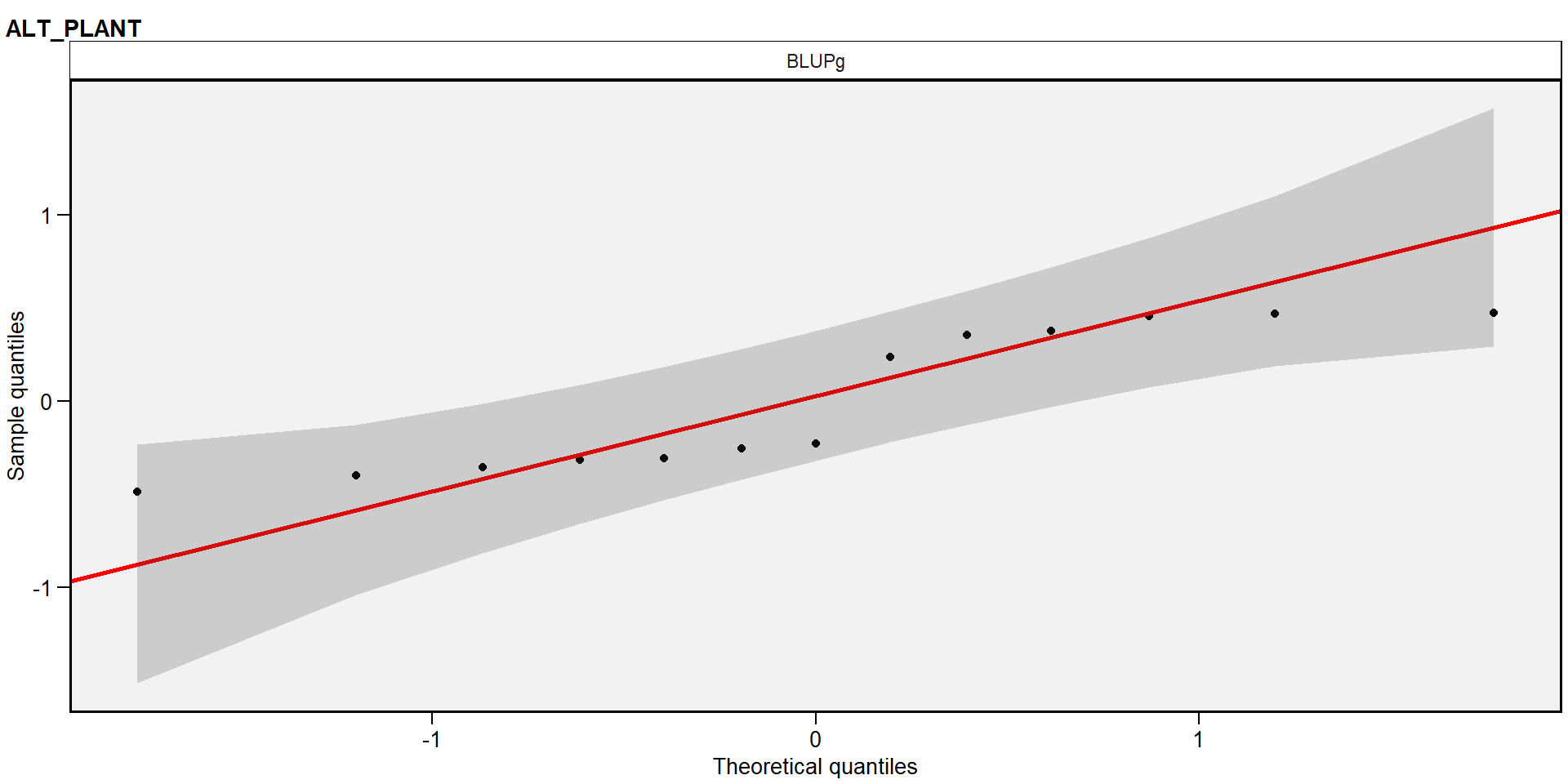
Detalhes da análise
details <- gmd(gen_mod, "details")
## Class of the model: gamem
## Variable extracted: details
print_tbl(details)
Parameters |
ALT_PLANT |
ALT_ESP |
COMPES |
DIAMES |
COMP_SAB |
DIAM_SAB |
MGE |
NFIL |
MMG |
NGE |
Ngen |
13 |
13 |
13 |
13 |
13 |
13 |
13 |
13 |
13 |
13 |
OVmean |
2.4619 |
1.3131 |
15.2333 |
48.7385 |
28.463 |
15.8874 |
168.4356 |
15.7949 |
333.8148 |
504.6513 |
Min |
1.814 (H8 in II) |
0.752 (H8 in II) |
12.5 (H12 in III) |
44.71 (H8 in I) |
23.852 (H8 in II) |
13.28 (H12 in III) |
105.7167 (H9 in I) |
13.2 (H10 in II) |
226.5956 (H9 in III) |
354 (H12 in II) |
Max |
3.04 (H3 in II) |
1.878 (H1 in I) |
17.94 (H6 in III) |
53.742 (H6 in III) |
33.018 (H2 in III) |
18.28 (H6 in III) |
236.1105 (H6 in III) |
18 (H13 in III) |
451.6832 (H3 in II) |
674.4 (H6 in III) |
MinGEN |
1.9593 (H8) |
0.846 (H8) |
13.36 (H12) |
45.2607 (H8) |
24.5707 (H8) |
13.88 (H12) |
112.3297 (H9) |
13.7333 (H11) |
236.2815 (H8) |
424 (H12) |
MaxGEN |
2.9467 (H2) |
1.7953 (H1) |
17.24 (H6) |
53.19 (H2) |
32.7087 (H2) |
17.62 (H6) |
218.8555 (H2) |
17.4667 (H2) |
415.6753 (H2) |
621.7333 (H6) |
LRT
lrt <- gmd(gen_mod, "lrt")
## Class of the model: gamem
## Variable extracted: lrt
print_tbl(lrt)
VAR |
model |
npar |
logLik |
AIC |
LRT |
Df |
Pr(>Chisq) |
ALT_PLANT |
Genotype |
4 |
-22.779 |
53.557 |
49.238 |
1 |
0.000 |
ALT_ESP |
Genotype |
4 |
-17.699 |
43.398 |
53.684 |
1 |
0.000 |
COMPES |
Genotype |
4 |
-66.845 |
141.690 |
13.617 |
1 |
0.000 |
DIAMES |
Genotype |
4 |
-91.660 |
191.320 |
24.927 |
1 |
0.000 |
COMP_SAB |
Genotype |
4 |
-89.278 |
186.555 |
32.902 |
1 |
0.000 |
DIAM_SAB |
Genotype |
4 |
-66.422 |
140.844 |
13.005 |
1 |
0.000 |
MGE |
Genotype |
4 |
-185.644 |
379.288 |
29.849 |
1 |
0.000 |
NFIL |
Genotype |
4 |
-68.094 |
144.188 |
10.145 |
1 |
0.001 |
MMG |
Genotype |
4 |
-202.400 |
412.800 |
28.734 |
1 |
0.000 |
NGE |
Genotype |
4 |
-202.803 |
413.607 |
6.808 |
1 |
0.009 |
Componentes de variância
vcomp <- gmd(gen_mod, "vcomp")
## Class of the model: gamem
## Variable extracted: vcomp
print_tbl(vcomp)
Group |
ALT_PLANT |
ALT_ESP |
COMPES |
DIAMES |
COMP_SAB |
DIAM_SAB |
MGE |
NFIL |
MMG |
NGE |
GEN |
0.155 |
0.118 |
1.205 |
5.992 |
5.698 |
1.154 |
1172.429 |
1.137 |
2941.365 |
1682.787 |
Residual |
0.013 |
0.008 |
0.734 |
1.703 |
1.043 |
0.739 |
252.595 |
0.941 |
673.583 |
2014.021 |
plot(gen_mod, type = "vcomp")
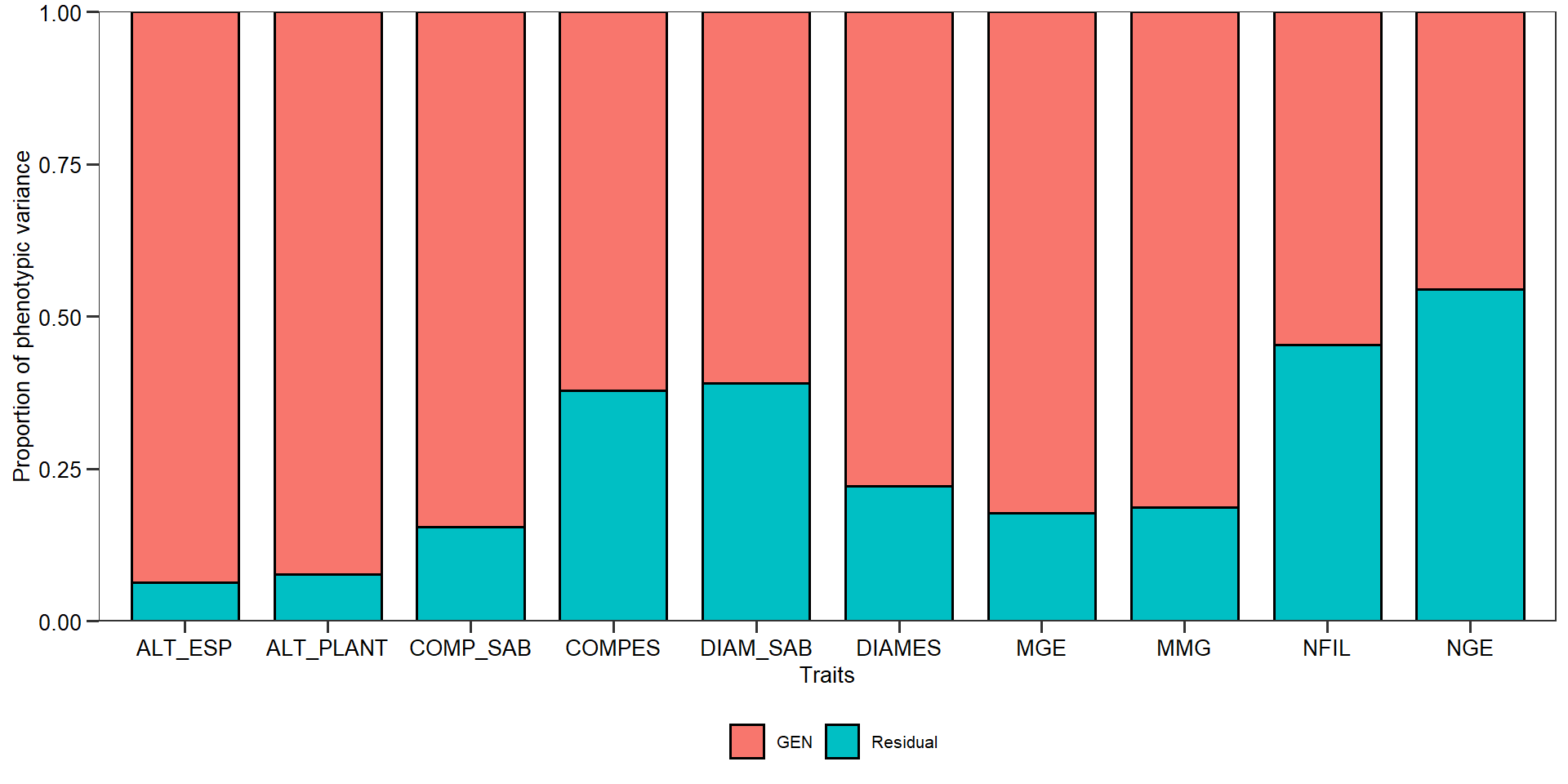
Parâmetros genéticos
genpar <- gmd(gen_mod, "genpar")
## Class of the model: gamem
## Variable extracted: genpar
print_tbl(genpar)
Parameters |
ALT_PLANT |
ALT_ESP |
COMPES |
DIAMES |
COMP_SAB |
DIAM_SAB |
MGE |
NFIL |
MMG |
NGE |
Gen_var |
0.155 |
0.118 |
1.205 |
5.992 |
5.698 |
1.154 |
1172.429 |
1.137 |
2941.365 |
1682.787 |
Gen (%) |
92.383 |
93.700 |
62.139 |
77.867 |
84.522 |
60.954 |
82.274 |
54.722 |
81.367 |
45.520 |
Res_var |
0.013 |
0.008 |
0.734 |
1.703 |
1.043 |
0.739 |
252.595 |
0.941 |
673.583 |
2014.021 |
Res (%) |
7.617 |
6.300 |
37.861 |
22.133 |
15.478 |
39.046 |
17.726 |
45.278 |
18.633 |
54.480 |
Phen_var |
0.168 |
0.126 |
1.939 |
7.695 |
6.741 |
1.894 |
1425.024 |
2.078 |
3614.948 |
3696.808 |
H2 |
0.924 |
0.937 |
0.621 |
0.779 |
0.845 |
0.610 |
0.823 |
0.547 |
0.814 |
0.455 |
h2mg |
0.973 |
0.978 |
0.831 |
0.913 |
0.942 |
0.824 |
0.933 |
0.784 |
0.929 |
0.715 |
Accuracy |
0.987 |
0.989 |
0.912 |
0.956 |
0.971 |
0.908 |
0.966 |
0.885 |
0.964 |
0.845 |
CVg |
15.983 |
26.209 |
7.205 |
5.022 |
8.386 |
6.762 |
20.329 |
6.751 |
16.247 |
8.129 |
CVr |
4.589 |
6.796 |
5.624 |
2.678 |
3.589 |
5.412 |
9.436 |
6.141 |
7.775 |
8.893 |
CV ratio |
3.483 |
3.857 |
1.281 |
1.876 |
2.337 |
1.249 |
2.154 |
1.099 |
2.090 |
0.914 |
BLUPs preditos
blupg <- gmd(gen_mod, "blupg")
## Class of the model: gamem
## Variable extracted: blupg
print_tbl(blupg)
GEN |
ALT_PLANT |
ALT_ESP |
COMPES |
DIAMES |
COMP_SAB |
DIAM_SAB |
MGE |
NFIL |
MMG |
NGE |
H1 |
2.917 |
1.785 |
15.017 |
51.603 |
31.340 |
15.563 |
186.872 |
16.792 |
385.023 |
490.175 |
H10 |
2.060 |
0.994 |
15.477 |
46.904 |
26.891 |
16.229 |
160.465 |
14.388 |
317.204 |
508.904 |
H11 |
2.155 |
1.025 |
15.139 |
47.439 |
27.304 |
15.696 |
163.931 |
14.179 |
341.393 |
485.600 |
H12 |
2.103 |
0.904 |
13.676 |
46.909 |
27.301 |
14.233 |
133.764 |
15.120 |
314.632 |
447.000 |
H13 |
2.234 |
1.112 |
15.627 |
50.133 |
31.760 |
16.617 |
169.027 |
16.583 |
327.351 |
517.672 |
H2 |
2.934 |
1.735 |
15.859 |
52.805 |
32.464 |
16.469 |
215.477 |
17.105 |
409.870 |
523.772 |
H3 |
2.928 |
1.740 |
15.294 |
50.207 |
29.987 |
15.865 |
189.434 |
15.120 |
395.348 |
483.122 |
H4 |
2.836 |
1.620 |
16.297 |
49.308 |
29.574 |
16.975 |
195.519 |
15.120 |
384.768 |
508.808 |
H5 |
2.698 |
1.418 |
16.270 |
48.200 |
27.479 |
16.821 |
185.049 |
15.956 |
332.910 |
544.502 |
H6 |
2.815 |
1.544 |
16.901 |
51.369 |
26.981 |
17.315 |
211.906 |
16.687 |
345.164 |
588.344 |
H7 |
2.145 |
1.113 |
14.712 |
47.449 |
27.260 |
15.568 |
145.452 |
15.642 |
295.030 |
492.081 |
H8 |
1.973 |
0.856 |
13.953 |
45.562 |
24.795 |
14.557 |
116.678 |
16.269 |
243.199 |
484.695 |
H9 |
2.206 |
1.223 |
13.809 |
45.714 |
26.883 |
14.629 |
116.089 |
16.374 |
247.701 |
485.791 |
# plotar os BLUPS (default)
plot_blup(gen_mod)
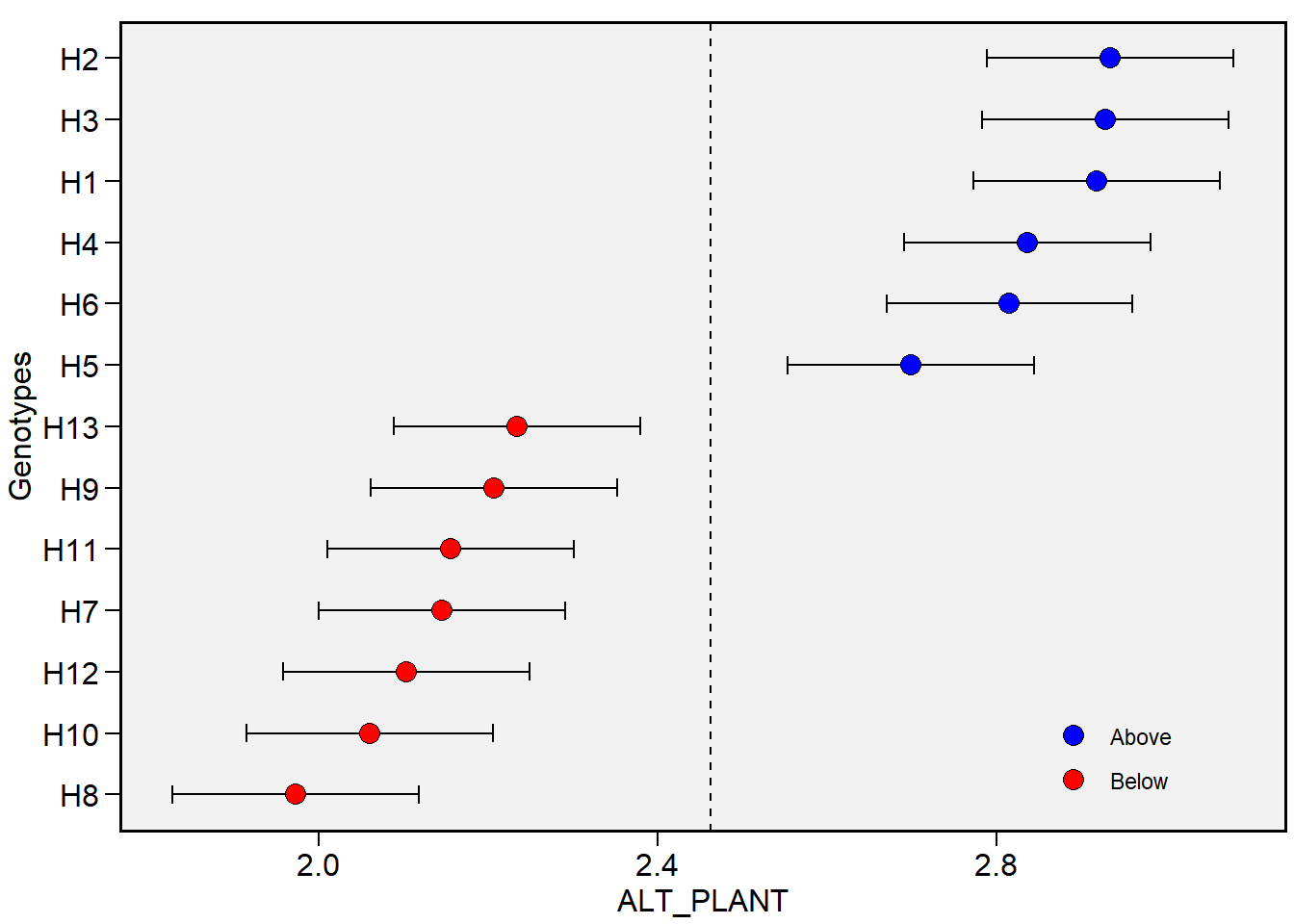
# Trait MGE
plot_blup(gen_mod,
var = "MGE",
height.err.bar = 0,
col.shape = c("black", "gray"),
x.lab = "Massa de grãos por espiga (g)",
y.lab = "Híbridos de milho")
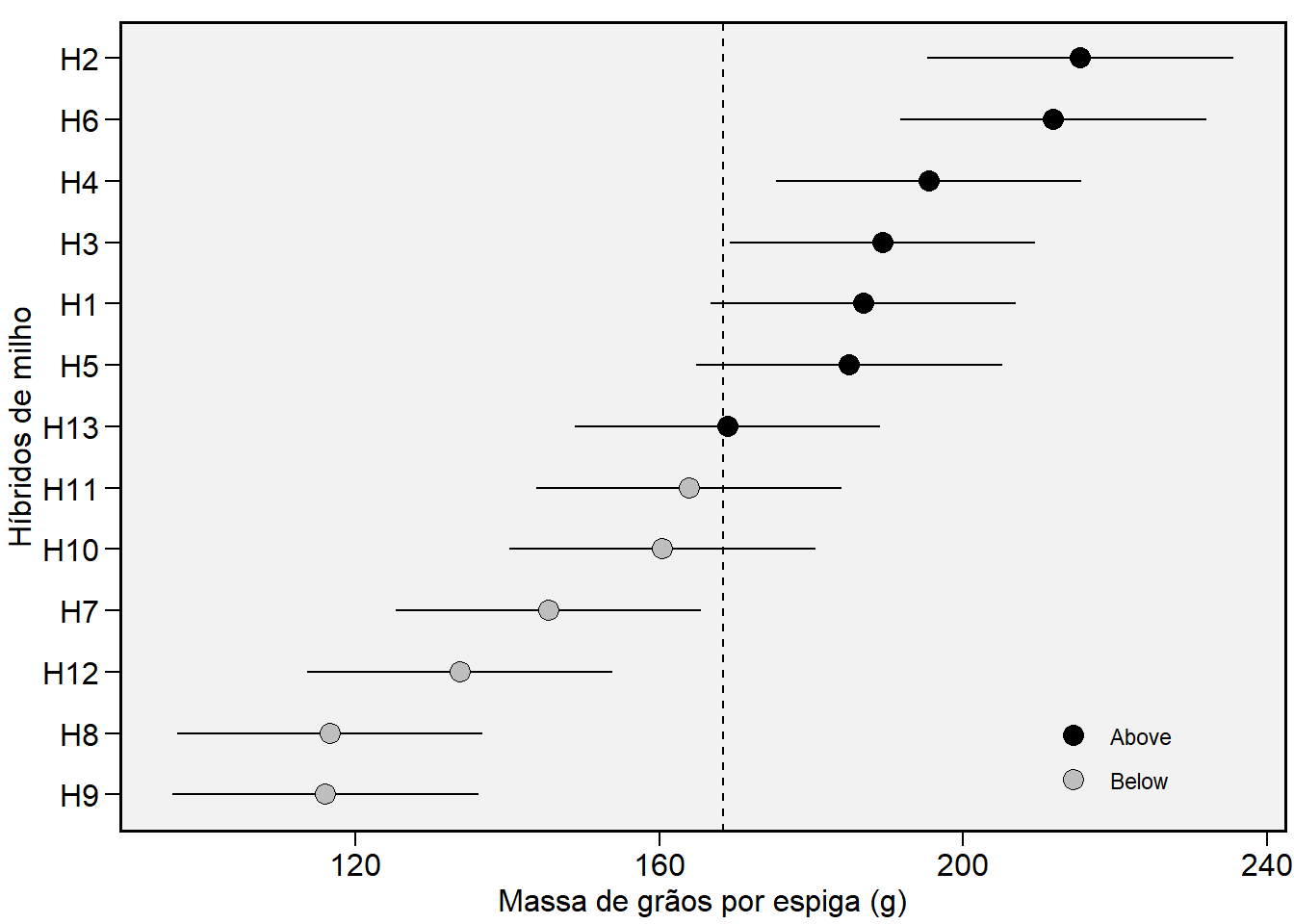
Modelos mistos - dentro de ambientes
df_ge <- import("http://bit.ly/df_ge", setclass = "tbl")
mod_gen_whithin <-
gamem(df_ge,
gen = GEN,
rep = BLOCO,
resp = everything(),
by = ENV, verbose = FALSE)
gmd(mod_gen_whithin, "lrt") %>% print_tbl()
ENV |
VAR |
model |
npar |
logLik |
AIC |
LRT |
Df |
Pr(>Chisq) |
A1 |
ALT_PLANT |
Genotype |
4 |
19.651 |
-31.302 |
0.232 |
1 |
0.630 |
A1 |
ALT_ESP |
Genotype |
4 |
18.480 |
-28.960 |
2.134 |
1 |
0.144 |
A1 |
COMPES |
Genotype |
4 |
-61.532 |
131.065 |
0.000 |
1 |
1.000 |
A1 |
DIAMES |
Genotype |
4 |
-77.909 |
163.817 |
7.960 |
1 |
0.005 |
A1 |
COMP_SAB |
Genotype |
4 |
-84.379 |
176.758 |
12.568 |
1 |
0.000 |
A1 |
DIAM_SAB |
Genotype |
4 |
-58.807 |
125.614 |
0.103 |
1 |
0.748 |
A1 |
MGE |
Genotype |
4 |
-162.635 |
333.270 |
2.073 |
1 |
0.150 |
A1 |
NFIL |
Genotype |
4 |
-80.166 |
168.333 |
2.552 |
1 |
0.110 |
A1 |
MMG |
Genotype |
4 |
-186.894 |
381.789 |
3.654 |
1 |
0.056 |
A1 |
NGE |
Genotype |
4 |
-205.051 |
418.101 |
0.529 |
1 |
0.467 |
A2 |
ALT_PLANT |
Genotype |
4 |
-22.779 |
53.557 |
49.238 |
1 |
0.000 |
A2 |
ALT_ESP |
Genotype |
4 |
-17.699 |
43.398 |
53.684 |
1 |
0.000 |
A2 |
COMPES |
Genotype |
4 |
-66.845 |
141.690 |
13.617 |
1 |
0.000 |
A2 |
DIAMES |
Genotype |
4 |
-91.660 |
191.320 |
24.927 |
1 |
0.000 |
A2 |
COMP_SAB |
Genotype |
4 |
-89.278 |
186.555 |
32.902 |
1 |
0.000 |
A2 |
DIAM_SAB |
Genotype |
4 |
-66.422 |
140.844 |
13.005 |
1 |
0.000 |
A2 |
MGE |
Genotype |
4 |
-185.644 |
379.288 |
29.849 |
1 |
0.000 |
A2 |
NFIL |
Genotype |
4 |
-68.094 |
144.188 |
10.145 |
1 |
0.001 |
A2 |
MMG |
Genotype |
4 |
-202.400 |
412.800 |
28.734 |
1 |
0.000 |
A2 |
NGE |
Genotype |
4 |
-202.803 |
413.607 |
6.808 |
1 |
0.009 |
A3 |
ALT_PLANT |
Genotype |
4 |
-0.947 |
9.893 |
3.810 |
1 |
0.051 |
A3 |
ALT_ESP |
Genotype |
4 |
3.562 |
0.877 |
0.561 |
1 |
0.454 |
A3 |
COMPES |
Genotype |
4 |
-55.480 |
118.959 |
0.073 |
1 |
0.786 |
A3 |
DIAMES |
Genotype |
4 |
-91.901 |
191.802 |
17.595 |
1 |
0.000 |
A3 |
COMP_SAB |
Genotype |
4 |
-86.207 |
180.414 |
22.367 |
1 |
0.000 |
A3 |
DIAM_SAB |
Genotype |
4 |
-52.489 |
112.979 |
2.449 |
1 |
0.118 |
A3 |
MGE |
Genotype |
4 |
-165.345 |
338.690 |
5.002 |
1 |
0.025 |
A3 |
NFIL |
Genotype |
4 |
-71.073 |
150.146 |
7.673 |
1 |
0.006 |
A3 |
MMG |
Genotype |
4 |
-190.433 |
388.866 |
6.716 |
1 |
0.010 |
A3 |
NGE |
Genotype |
4 |
-205.860 |
419.720 |
6.723 |
1 |
0.010 |
A4 |
ALT_PLANT |
Genotype |
4 |
9.295 |
-10.590 |
0.000 |
1 |
1.000 |
A4 |
ALT_ESP |
Genotype |
4 |
13.658 |
-19.316 |
0.000 |
1 |
1.000 |
A4 |
COMPES |
Genotype |
4 |
-65.282 |
138.564 |
6.803 |
1 |
0.009 |
A4 |
DIAMES |
Genotype |
4 |
-83.220 |
174.441 |
0.235 |
1 |
0.628 |
A4 |
COMP_SAB |
Genotype |
4 |
-76.511 |
161.022 |
4.359 |
1 |
0.037 |
A4 |
DIAM_SAB |
Genotype |
4 |
-62.685 |
133.371 |
3.074 |
1 |
0.080 |
A4 |
MGE |
Genotype |
4 |
-174.257 |
356.514 |
0.095 |
1 |
0.758 |
A4 |
NFIL |
Genotype |
4 |
-65.683 |
139.366 |
1.401 |
1 |
0.237 |
A4 |
MMG |
Genotype |
4 |
-183.409 |
374.818 |
1.755 |
1 |
0.185 |
A4 |
NGE |
Genotype |
4 |
-210.085 |
428.170 |
0.966 |
1 |
0.326 |
gmd(mod_gen_whithin, "vcomp") %>% print_tbl()
ENV |
Group |
ALT_PLANT |
ALT_ESP |
COMPES |
DIAMES |
COMP_SAB |
DIAM_SAB |
MGE |
NFIL |
MMG |
NGE |
A1 |
GEN |
0.001 |
0.004 |
0.000 |
1.756 |
3.085 |
0.068 |
100.060 |
1.139 |
512.906 |
524.620 |
A1 |
Residual |
0.015 |
0.013 |
1.443 |
1.828 |
2.050 |
1.173 |
296.826 |
2.925 |
1014.606 |
3663.805 |
A2 |
GEN |
0.155 |
0.118 |
1.205 |
5.992 |
5.698 |
1.154 |
1172.429 |
1.137 |
2941.365 |
1682.787 |
A2 |
Residual |
0.013 |
0.008 |
0.734 |
1.703 |
1.043 |
0.739 |
252.595 |
0.941 |
673.583 |
2014.021 |
A3 |
GEN |
0.017 |
0.005 |
0.047 |
5.369 |
4.269 |
0.240 |
180.972 |
1.181 |
840.939 |
1982.321 |
A3 |
Residual |
0.033 |
0.034 |
0.984 |
2.430 |
1.415 |
0.634 |
280.409 |
1.271 |
1018.430 |
2398.781 |
A4 |
GEN |
0.000 |
0.000 |
0.809 |
0.398 |
1.216 |
0.474 |
39.561 |
0.375 |
291.452 |
944.826 |
A4 |
Residual |
0.028 |
0.022 |
0.969 |
4.417 |
2.101 |
1.065 |
717.405 |
1.442 |
967.158 |
4595.334 |